The Future of Healthcare - Improving Health Service Delivery Through Digital Innovation
- monachalla
- Mar 30, 2021
- 7 min read
Updated: Apr 6, 2021
As our public demographic evolves and grows so too do our public service needs. This increase in demand requires an expansion of infrastructure which introduces extra costs. As a result, digital tools are introduced as a cost-saving solution to help alleviate pressure on human resources. The Government of Canada has started to use tools such as Artificial Intelligence (AI) to increase efficiency and quality of public service delivery (Government of Canada, 2020a). But what is AI? The Canadian Institute for Advanced Research (CIFAR) defines it as “any current or future machine learning approach to predictive analytics, decision-support systems and/or automated decision-making” (CIFAR, 2020). Overtime, the use of AI has been increasing, and it continues to play a major role in contributing to digitally innovative solutions (CIFAR, 2020). Furthermore, the use of such innovative solutions not only helps reduce public expenditures but also helps improve access to government services, resulting in better public resource equity. However, as with any new tool, there are challenges that arise. This discussion will highlight the benefits of AI in public service delivery, but also explore some risks governments may face when incorporating AI into these delivery frameworks, and what measures can be taken in order to reduce or avoid these risks.
For the purposes of this discussion, I will be focusing on the use of AI in healthcare. Many countries today are facing an increasing cost of healthcare, and AI is presenting a possible solution to this problem (Clark, 2019). A study by Deloitte highlights that the documenting and recording of information alone costs more than $16 billion in wages, a cost that could be reduced or eliminated by introducing administrative AI systems (Araya, 2019). However, AI not only has the potential to save costs, but also increase the quality of care patients receive and decrease the time patients spend waiting for results (Clark, 2019). By decreasing wait times this allows for early diagnoses leading to lower treatment costs and improved treatment outcomes (Clark, 2019). The AI4Health Task Force emphasized that Canada’s greatest opportunity is in the development of a strategy and collaborative vision for AI in health (Strome, 2020). Deloitte captures the potential AI in health can have on the individual and how we utilize health information to enhance data collection and service delivery.
The Canadian government has begun to understand the importance of carefully considering how social determinants of health are influencing health outcomes in Canada. The federal government identifies access to health services as a determinant of health, this determinant can be influenced by factors such as rurality, socioeconomic status, and residency/immigration status (Government of Canada, 2020b; Hajizadeh, 2017). A study by Hajizadeh (2017) highlights that refugees migrating to Canada have little input regarding their settlement location and are often placed in rural communities. Unfortunately, these communities generally lack access to specialized health services, such as 3D ultrasound, which is widely used for diagnosis and monitoring of cardiovascular disease, pregnancy, vascular issues, and other soft tissue imaging (CIFAR, 2020). Hajizadeh (2017) also concludes that individuals from low socioeconomic backgrounds also live in communities where access to health services are limited. However, an Edmonton-based start-up, MEDO.ai, utilized AI and cloud-computing to bring 3D ultrasound to these underserved communities (CIFAR, 2020). The system improves the accessibility of ultrasound services by using a portable imaging device and using AI-based analysis, therefore enabling individuals to access these specialized healthcare services from within their communities (CIFAR, 2020). This digital solution improves the accessibility of this healthcare service by eliminating exhaustive travel needs and reducing service wait-times (CIFAR, 2020).
Social determinants of health help identify factors that influence the health of individuals. However, models such as the socio-ecological model help organize these determinants into distinct spheres, helping governments design appropriate policies and programs to address health issues (Thompson et al., 2015). However, having distinct solutions for each sphere of health may give rise to a complex health system, making it difficult for individuals to navigate. Digitalizing healthcare services can facilitate user navigation by creating a central system for health resources. In Nova Scotia, the provincial government is working towards implementing a One Patient One Record health information system. This system would help health professionals access a full range of their patient’s health information, facilitating their ability to provide quality care (Health and Wellness, 2016). Since patients’ information would already be stored within this system, to optimize the system’s potential patients could be given access to their specific file and AI processes could be used to consolidate a collection of resources that benefit the patient. Ultimately, this would help patients, especially those part of vulnerable populations or those with poor social-networks, to navigate healthcare services, from acquiring health education, to connecting with community groups, to accessing government grants.
Much of the anxiety about AI in health stems from public concern of the “dehumanization” of healthcare (Clark, 2019). Healthcare is known for its complexity, health professionals undergo extensive training to learn to be cognisant of their inherent biases and to demonstrate cultural competency. Health professionals are self-reflective and expected to learn from their social interactions and know that each patient’s case is slightly unique and must be treated as such. The problem with AI is that once an algorithm is created an AI system will run the same program on each patient – which may be inappropriate. In his presentation, Haas (2017) draws attention to the Compass Criminal Sentencing Algorithm, an AI-based system used by judicial systems in 13 states in America. The algorithm determines the risk a convict would commit another crime once they are released, this data is used to set bail. The greater the risk of reoccurring crime, the greater the bail was set (Haas, 2017). An article by ProPublica noted that the system was 77% more likely to target African-American inmates (Haas, 2017). Translated to the healthcare system, many studied have concluded that the presentation of cardiovascular disease differs between Black and White patients (Chaturvedi, 2003; Hackler et al., 2019). If AI health systems are designed to only recognize symptoms more prominent in White patients, this leaves Black patients vulnerable to misdiagnosis or no diagnosis, resulting in systemic injustices in health service delivery (Chaturvedi, 2003; Hackler et al., 2019).
Nonetheless, AI does enhance the accessibility of healthcare services. Recognizing its uses, but also realizing its limitations, AI in health is currently best applied when used as an AI-augmented system to support healthcare service delivery, thus improving access to health services and reducing infrastructure-related barriers (Clark, 2019). AI-augmentation helps healthcare systems optimize their potential and save on costs, while also ensuring that the humanistic aspect of healthcare is not lost. Augmentation of government and government services through AI is critical to the modernization of the public sector (Araya, 2019).
On top of using AI as an augmented system, it is also important to be critical of the algorithms being used. This can be supported by ensuring AI health systems are transparent and their outcomes reported (Government of Canada, 2020a). The Government of Canada’s AI procurement strategy therefore emphasizes the importance of agility, transparency, and collaboration. The Government of Canada highlights the importance of understanding and measuring the impact of AI technologies and being transparent about where and how AI is being used in government, as well as sharing AI source code with the public (Government of Canada, 2020a). The Canadian government also discusses the importance of ensuring AI decision-making and outcomes are explainable to the public (Government of Canada, 2020a). Finally, the Government of Canada highlights the importance of training those developing and using AI technology to ensure the systems they create do not present biases (Government of Canada, 2020a). By guaranteeing these expectations of AI systems are met, this enables the public to better understand AI, trust it, and be able to hold governments accountable for the outcomes of AI if necessary.
In conclusion, digital innovation such as AI is a powerful tool that can help advance governments and the services they provide. AI in health can not only improve the cost-effectiveness of healthcare systems, but it can also improve the accessibility of healthcare services, resulting in resource equity. However, it is important to be cognisant of the limitations of AI in healthcare and to be critical of its function (Haas, 2017), because while AI can provide significant advantages in health, failure could deter institutions from integrating AI into their systems (Van Buren, Chew, & Eggers, 2020). If not designed ethically or just, AI can have negative consequences on the health of minority, vulnerable, or all populations. In order to avoid these risks, there are two key solutions: (1) AI-augmentation to support health professionals and enhance their quality of care, and (2) ensure AI systems are agile, transparent, and collaborative so that the public can hold these systems and their designers accountable. It is not the use of AI technology that presents a risk to healthcare or the public sector, but would be the laziness of those designing and introducing these systems into the public service (Haas, 2017).
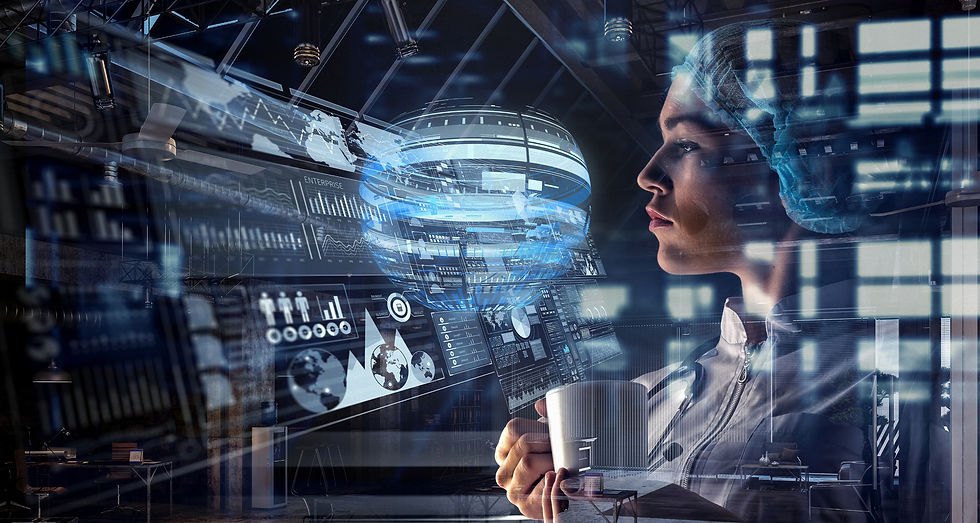
References:
Araya, D. (2019, January 15). Artificial Intelligence And The End Of Government. Retrieved November 23, 2020, from https://www.forbes.com/sites/danielaraya/2019/01/04/artificial-intelligence-and-the-end-of-government/?sh=6ba36ac719b4
Chaturvedi N. (2003). Ethnic differences in cardiovascular disease. Heart (British Cardiac Society), 89(6), 681–686. https://doi.org/10.1136/heart.89.6.681, from https://www.ncbi.nlm.nih.gov/pmc/articles/PMC1767706/
CIFAR. (2020, July). Building a Learning Health System for Canadians. Retrieved November 22, 2020, from https://www.cifar.ca/docs/default-source/ai-reports/ai4health-report-eng-f.pdf
Clark, M. (Ed.). (2019, November 03). AI for Good: Paths forward. Retrieved November 22, 2020, from https://www.itu.int/en/itunews/Documents/2019/2019-03/2019_ITUNews03-en.pdf
Government of Canada (a). (2020, July 28). Responsible use of artificial intelligence (AI). Retrieved November 23, 2020, from https://www.canada.ca/en/government/system/digital-government/digital-government-innovations/responsible-use-ai.html
Government of Canada (b). (2020, October 07). Social determinants of health and health inequalities. Retrieved April 06, 2021, from https://www.canada.ca/en/public-health/services/health-promotion/population-health/what-determines-health.html
Hackler, E., Lew, J., Gore, M. O., Ayers, C. R., Atzler, D., Khera, A., . . . De Lemos, J. A. (2019). Racial Differences in Cardiovascular Biomarkers in the General Population. Journal of the American Heart Association, 8(18). doi:10.1161/jaha.119.012729, from https://www.ahajournals.org/doi/10.1161/JAHA.119.012729
Hajizadeh, M. (2017). Does socioeconomic status Affect lengthy wait time in Canada? Evidence from CANADIAN community Health Surveys. The European Journal of Health Economics, 19(3), 369-383. doi:10.1007/s10198-017-0889-3, from https://link.springer.com/article/10.1007/s10198-017-0889-3
Health and Wellness. (2016, December 06). One person one Record project moves to next phase. Retrieved April 06, 2021, from https://novascotia.ca/news/release/?id=20161206001
Munro, D. (2019, January 11). Navigating the risks and rewards of governing AI. Retrieved November 23, 2020, from https://policyoptions.irpp.org/fr/magazines/january-2019/navigating-the-risks-and-rewards-of-governing-ai/
Mozur, P., Zhong, R., & Krolik, A. (2020, March 02). In Coronavirus Fight, China Gives Citizens a Color Code, With Red Flags. Retrieved November 03, 2020, from https://www.nytimes.com/2020/03/01/business/china-coronavirus-surveillance.html
Strome, E. (2020, September 25). An AI for Health strategy will be a gamechanger for Canada. Retrieved November 23, 2020, from https://www.cifar.ca/cifarnews/2020/09/25/an-ai-for-health-strategy-will-be-a-gamechanger-for-canada
The Real Reason to be Afriad of Artificial Intelligence | Peter Haas | TEDx Talks [Video file]. (2017, December 15). Retrieved November 17, 2020, from https://www.youtube.com/watch?v=TRzBk_KuIaM&feature=emb_logo
Thompson, N. J., McGee, R., Walker, E., & Munoz, L. (2015). Reflections on mental health advocacy across differing ecological levels. Journal of the Georgia Public Health Association, 5(1). doi:10.20429/jgpha.2015.050131
Van Buren, E., Chew, B., & Eggers, W. D. (2020, January 24). AI readiness for government. Retrieved November 23, 2020, from https://www2.deloitte.com/us/en/insights/industry/public-sector/ai-readiness-in-government.html
Comments